What significant advancements can be achieved by incorporating AI technology into nanoparticle tracking imaging technology?
Recently, Professor Fang Ning's team at Xiamen University has developed an automated, high-speed, and multidimensional single particle tracking (SPT) system based on deep learning, breaking through the limitations of nanoparticle rotation tracking in the cellular microenvironment.
Deep learning can learn and extract features from complex images and sequence data, thereby enabling all-around, precise tracking of single molecules or individual nanoparticles within living cells at the nanoscale. Not only has it tracked the displacement in three-dimensional space, but for the first time, it has also observed the rotational motion of molecules/nanoparticles.
It is important to understand that in the field of biomedicine, controlling the rotational motion of nanoparticles can achieve manipulation and detection of cells and biomolecules. In fact, the rotational motion of nanoparticles is closely related to many important biological processes, such as serving as probes in biosensors, being used for nano-drug delivery, and elucidating the mechanisms of entry into cells.
This method has a wide range of applications in imaging research, such as exploring the rotational and translational behaviors of probes in complex environments. Under the condition of sample preparation completion, it can achieve an automated process from instrument data processing to analysis, laying the foundation for the future realization of a complete automated drug delivery process, and providing a new solution for high-speed screening of drug delivery research and pharmaceutical production lines.The reviewer commented on the study, stating: "The authors introduced an automated, high-speed, multidimensional SPT system for tracking the three-dimensional orientation of anisotropic gold nanoprobes within living cells. In particular, they provided a detailed explanation of the construction and preprocessing of the dataset for training the Convolutional Neural Network (CNN). Through this method, they demonstrated the ability to train an effective model."
Advertisement
Recently, the related paper was published under the title "Deep Learning-Assisted Automated Multidimensional Single Particle Tracking in Living Cells" in Nano Letters[1].
Xiamen University doctoral students Dongliang Song and Xin Zhang are the co-first authors, and Professor Ning Fang from Xiamen University, Dr. Xiaodong Cheng from Wenzhou Medical University, and Senior Researcher Jie Ming Li from Bristol-Myers Squibb Company are the co-corresponding authors.
Tracking eight dimensions of information in living cells simultaneously
Traditional single-particle tracking methods can usually only handle two-dimensional plane or time series data. Under normal experimental conditions, due to the extremely small size of the nanoparticle, the signal is weak in the cellular environment or tissue environment, and it is impossible to see its rotation process. Moreover, the relevant signals obtained by theoretical analysis are not only inefficient but also prone to human error.Fang Ning pointed out, "If we can detect the trend of changes in the probe's restricted process in real time, we can know what specific restrictions it is facing."
To address the aforementioned issue, the research team has developed an automated, high-speed, multi-dimensional SPT system integrated with deep learning algorithms, which is used to track the 3D orientation of anisotropic gold nanoparticle probes in living cells with high positioning accuracy (<10nm) and time resolution (as fast as 0.9ms), overcoming the limitations of rotational tracking under low signal-to-noise ratio conditions.
The research team introduced AI into complex experiments to help and replace the previous manual intervention and judgment processes, automatically recognizing and classifying the motion processes in complex cellular environments, tracking target probes, and directly analyzing the mechanism of drug delivery particles.
In SPT experiments, by revealing the translational and rotational dynamics of anisotropic optical nanoprobes, it is possible to provide molecular-level information about cellular activities.
This method can solve the azimuth angle (0°-360°) and polar angle (0°-90°) on experimental and simulated data with a signal-to-noise ratio of about 4, with an error of less than 2°. Even when the signal-to-noise ratio approaches the limit of 1, this method still maintains better robustness and noise resistance than traditional pattern matching methods.It is noteworthy that in this study, researchers have achieved multi-dimensional imaging including space, time, and fluorescence intensity. Fang Ning pointed out, "This experiment is the most complex cell imaging experiment to date, and we have achieved simultaneous imaging in eight dimensions."
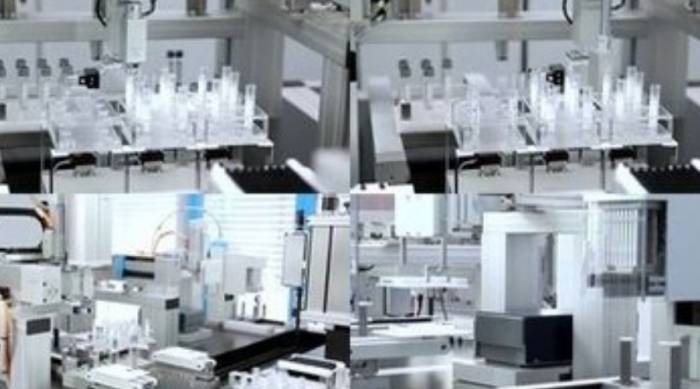
Through experiments on the drug delivery process along microtubules inside living cells, the effectiveness of the multi-dimensional SPT (Single-Particle Tracking) system was demonstrated. The research involved a large amount of interdisciplinary integration, including optical imaging, hardware construction, AI algorithm design, and program data analysis. "In this study, Song Dongliang did a lot of work and integrated various disciplines," said Fang Ning.
Promoting the development towards single-molecule diagnosis and treatment, drug delivery, and precision medicine
According to the introduction, the team divides the introduction of AI into experiments into three stages: automatic recognition of images, classification of motion patterns and cell behavior, and prediction.In the study, the first phase has been completed, which is the automatic image recognition based on data generated by computational simulation. Currently, they are about to complete the second phase, which is to track the cellular processes and classify them according to motion patterns.
Fang Ning gave an example: "For instance, the delivery of nanomedicine in the cell passes through the cell membrane and is transported to the final target location. The release process goes through different stages, and thus its molecular behavior is also different. The goal of this phase is to be able to automatically recognize the classification of cellular motion."
In the second phase, in addition to image recognition, a large amount of information judgment is also needed, so deep learning is required to take the entire biological process in the cell as the training set. It is reported that the research group is currently using the data from the laboratory over the past decade for training.
The third phase is based on the classification of motion patterns to predict the progress and results of drug delivery. Fang Ning said: "After obtaining a large amount of data through various microscopes or electron microscopes, we can analyze large-scale datasets and real-time dynamics of living cells through AI, which is very meaningful for the pharmaceutical industry."
At present, although there are many related applications of molecular diagnosis in China, the tracking of single molecules using imaging technology is still in a blank stage.Abroad, the field of single-molecule medical diagnosis and treatment has already seen companies entering the commercialization phase. In 2022, the American single-molecule tracking company Eikon Therapeutics secured more than 500 million U.S. dollars in financing.
At this stage, the direct product of this technology is scientific instruments, for which researchers have previously applied for relevant international patents. It is reported that in the future, the team plans to integrate more data related to the drug delivery systems of pharmaceutical companies, to include the process of drug efficacy evaluation and the entire process.
From the perspective of scientific instruments, this instrument method has already had a significant impact in the field of single-molecule/single-particle imaging. "I hope that this technology is not only confined to the laboratory, but also promotes the development towards new directions such as single-molecule diagnosis and treatment, nano-drug delivery, and precision medicine. We are also promoting related cooperation," said Fang Ning.
"After going through thousands of sails, I still come back as a young man."Fang Ning graduated from Xiamen University with a bachelor's degree, and after obtaining his Ph.D. from the University of British Columbia in Canada, he engaged in postdoctoral research at the Ames National Laboratory of the U.S. Department of Energy and Iowa State University. Subsequently, he served as an assistant professor at Iowa State University and a professor at Georgia State University in the United States.
In 2021, Fang Ning joined his alma mater, Xiamen University, as a Distinguished Professor and Xianghua Chair Professor on a full-time basis. His current team consists of more than 30 members, focusing on research directions such as biological and chemical optical imaging, including the development and application of optical imaging instruments, single-particle rotation tracking technology, and single-molecule chemical imaging.
Regarding his return to serve in his home country, Fang Ning expressed his feelings: "Although the research conditions in the United States are good in all aspects, I believe that returning to my motherland before the age of 45 is a golden opportunity, and there is still a lot of time to do more things. Returning to my alma mater, Xiamen University, is an honor for me."
Previously, Fang Ning led his research team to address the problem of measuring the dynamic process of key biomolecular conformational transformations in living cells, which was difficult to achieve. For the first time, they resolved a dispute in the field through experiments. They developed a brand-new single-particle rotation tracking system and were the first to observe the directional rotation of vesicles along the chiral direction of the kinesin protein helix in living cells, making a breakthrough in elucidating the molecular mechanism of receptor-mediated endocytosis [2].
Professor Ludger Johannes of the German National Academy of Sciences and the Curie Institute in Paris, France, commented on the aforementioned research in Nature Cell Biology: "This method represents a technical masterpiece," and specifically pointed out, "In addition to contributing to the understanding of the molecular mechanism of kinesin function in the cellular context, the current research more generally highlights the power of angle-sensitive microscopy techniques and the greater contributions these techniques may make in the field of membrane biology [3]."To dynamically monitor the spatiotemporal distribution of proteins and interactions between proteins, the team developed a single-particle tracking technology with automatic three-dimensional focusing, high spatial and angular resolution. By theoretically deriving the semi-planar point spread function, they simulated the defocused patterns of single particles related to angles to resolve three-dimensional angles, and analyzed the rotational dynamics of vesicles during transport in cells in real time, discovering a new mode of interaction between motor proteins, vesicles, and cellular microtubules [4].
Yale E. Goldman, a member of the National Academy of Sciences and a professor at the University of Pennsylvania, pointed out in the Biophysical Journal: "This intelligent optical and analytical method can be directly applied to other intracellular materials, or used by other laboratories for high-resolution spatial angle imaging. Future research in this area is expected to reveal the puzzling relationship between the activities of molecular motors and the targeted transport of intracellular cargo [5]."
Cheng Xiaodong said that this new research is the first step in studying the life processes of living cells using deep learning/AI-assisted imaging. In the future, he hopes that with the help of AI, multi-dimensional imaging technology can be used to analyze and predict protein functions and cell behaviors.
Similar to "driving a car with autonomous driving perception capabilities," he said, "when the probe moves in the cell, it can analyze and judge the surrounding environment based on its behavior and predict the possibilities of the next step."
Fang Ning believes that with the support of AI technology, this method will promote further development of experiments and instruments. "Originally, only scientific research laboratories could afford and use them. In the future, we will commercialize the technology and expand it to the pharmaceutical field."
POST A COMMENT