Recently, researchers from Columbia University in the United States and the Sloan-Kettering Cancer Center have developed a new spatial transcriptomics analysis tool called Starfysh. It can analyze the spatial heterogeneity of gene expression in tissues under the premise of cross-patient samples.
As a spatial transcriptomics analysis tool, Starfysh has been open-sourced by the research team. At the same time, the team has also provided a code calling tutorial ().
Starfysh solves the shortcomings of low spatial resolution in some sequencing technologies currently available. This method can combine spatial transcriptome data of different tissues and corresponding histological images, and predict the proportion of cell types and cell states at different sites through spatial gene expression and histological features.
Compared with other spatial transcriptomics methods, Starfysh does not require single-cell data as a reference, so it has better flexibility in analysis, providing a new method for analyzing rare and difficult-to-match single-cell sequencing data.
For a large number of patient data, Starfysh has strong fusion capabilities, so it can perform large-scale comparative analysis, thus providing a comprehensive and in-depth analysis method.As a novel computational toolkit, Starfysh utilizes a variational inference-based approach to integrate histological images with spatial transcriptomics data, thereby performing deconvolution operations.
Advertisement
Due to the absence of the need for reference data from single-cell RNA-seq, this represents a significant advancement.
This is because, within histological images, there is information regarding spatial dependency structures that can guide the deconvolution of data, making this method more accurate than modeling approaches that target non-informative spatial-related error terms.
For most spatial techniques previously, they lacked true single-cell resolution. With the aid of a machine learning framework, histology and spatial transcriptomics can be combined, allowing for more refined discoveries from spatial transcriptomics.
Additionally, Starfysh can integrate spatial data from different studies and patient populations, thereby enabling the use of more patient samples to better identify rare tumor microenvironments or recognize drug resistance mechanisms in different tumor types.Currently, a considerable number of users and collaborators are already utilizing Starfysh. Scholars have even conducted a study on small frozen clinical specimens of melanoma with its assistance, and the related paper has been published in Nature Genetics.
The application of Starfysh is not limited to different types of tumor samples; it can also bring extensive applications to samples related to developmental biology or drug development. In the paper, the research team also listed some public data application effects in the mouse cerebral cortex.
At the same time, the team focused on metaplastic breast cancer in their research. With the help of Starfysh, they discovered special spatial properties that could provide guidance for the development of new treatment methods for metaplastic breast cancer.
In the meantime, some external collaborators are also actively exploring corresponding immune cell therapies in the hope of finding effective ways to treat this rare type of breast cancer. Overall, Starfysh will play a broad role in the field of biomedical data analysis.The Analysis of Spatial Gene Expression is in Urgent Need of a "Breakthrough"
It is understood that there are certain differences in the types and behaviors of cells at different locations within the human body. The spatial position of cells, as well as the interactions between cells, can play a decisive role in life activities, such as determining the development of tissues, determining the response to drugs, and determining the occurrence and prognosis of tumors.
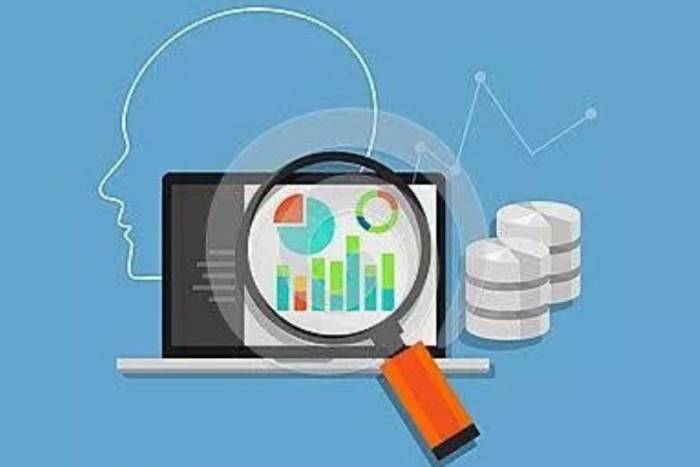
Previously, existing methods for analyzing spatial gene expression had certain limitations. For example, spatial transcriptome sequencing technology can only measure a limited number of genes; whole-genome spatial transcriptome sequencing technology has relatively low spatial resolution.
At the same time, whole-genome spatial transcriptome sequencing technology often cannot be used to assess a single type of cell, such as distinguishing the interactions between different immune cells at a certain site (spots) and the surrounding tissue.
Moreover, it is also unable to know the distribution of different cell types in space, so it can only conduct a general analysis at the level of gene expression with low spatial resolution.For understanding and dissecting complex biological systems, such as dissecting the tumor microenvironment, the aforementioned limitations pose certain obstacles.
For instance, breast cancer has different subtype classifications. When characterizing the microenvironment for different subtypes, it is necessary to describe and compare the types of cells in the tissue space, as well as analyze the reasons for different responses to therapy, such as analyzing the behavioral differences of immune cells among subtypes.
The pentagon inspires the name.
The reason for developing Starfysh is that researchers found that existing spatial data analysis methods rely on single-cell data. Therefore, they hope to develop a method that does not require single cells.At the beginning of the study, they first developed a semi-supervised learning algorithm, attempting to use bottleneck features to distinguish between cell types. However, the robustness and interpretability of this algorithm were relatively poor.
Then, the research group decided to incorporate cellular markers and other cellular characteristics, as well as Archetypes as prior knowledge. On the other hand, since visium data can match corresponding histological images, they began to consider how to utilize this part of the spatial information.
After multiple attempts with different models, they adopted the product of experts method to integrate images with spatial data. At the same time, they used auxiliary deep generative models to model the images and spatial omics.
In addition, the research group also used the Archetypes analysis method to obtain the prior distribution, thereby avoiding dependence on single-cell data.
Later, they adjusted the model again, no longer using the auxiliary deep generative model, but instead slightly modifying the data generation process to achieve better interpretability of cell types.When developing the toolkit, due to the need for validation on different datasets, they designed synthetic data and also utilized publicly available datasets.
In addition, the research group is very focused on samples of breast cancer of the type chemo-bio, hence the necessity to design simple and understandable tutorials to facilitate use by other researchers.
This requires them not only to have the capability of algorithm development but also to master the biomedical background knowledge involved in different types of data. Fortunately, the members of this team happen to have diverse backgrounds, which can bring a "cross-disciplinary boost" to the research.
In principle, Starfysh combines Archetypes analysis with graph probability model to simulate the sequencing process of gene expression.
Through Archetypes analysis, the most distinctive spots can be identified, which, together with the markers of known cell types, can help determine the expected cell types in the tissue or new cell types, and further find the special cell states present in a patient's sample.In the study, the research team used Starfysh to discover the spatial characteristics of interactions between tumor cells and various immune cells.
This difference is not only present in different subtypes of breast cancer, but may also be the reason for the drug resistance of certain subtypes, such as metaplastic breast cancer. Formulating new treatment plans based on this may effectively overcome drug resistance.
At the same time, the team focused on studying the tumor samples of metaplastic breast cancer and the differences with other triple-negative breast cancer samples.
The results showed that immune-suppressive cells, such as regulatory T cells, exhibit infiltration in metaplastic breast cancer, which helps cancer cells better adapt to the hypoxic microenvironment.
Researchers also found that Starfysh can be used for other related spatial data, such as characterizing and analyzing mouse lymph node tissue, human cerebral cortex tissue, and prostate cancer, etc.It can be seen that Starfysh is an effective method for analyzing the spatial characteristics of complex biological tissues and comprehensive atlases.
It is also reported that the research team initially verified the model on a synthetic dataset of three cell types. However, the latent variable of spots is continuous, so it appears as a triangle in low-dimensional visualization.
When there are five cell types, a starfish-like pentagonal shape appears directly. Although this pentagonal image was not used in the main figure of the paper, they took inspiration from it and named it Starfysh.
Finally, the related paper was published in Nature Biotechnology with the title "Starfysh integrates spatial transcriptomic and histologic data to reveal heterogeneous tumor-immune hubs" [1].
Doctoral students He Saiyu, Jin Yinuo, and Achille Nazaret are co-first authors. Professor Alexander Y. Rudensky and Professor George Plitas from the Sloan-Kettering Cancer Center in the United States, as well as Professor Elham Azizi from Columbia University in the United States, are co-corresponding authors.Researchers concluded by stating: "At present, AI for healthcare is becoming an increasingly popular topic, with tremendous potential for application in genomics and other biomedical fields. This study is a concrete example of the application of AI in biomedical data, and it is believed that there will be more and broader applications in the future."
POST A COMMENT