Recently, a team from Stanford University in the United States has utilized computer vision technology in deep learning to create a new deep learning model.
Starting from images of underground geological structures, they successfully predicted ground displacement caused by Carbon Capture and Sequestration (CCS) technology.
Compared to numerical simulation, this method can greatly improve the efficiency of prediction with a slight loss of accuracy, making it more economical and efficient.
In addition, the trained deep learning model can also be extended to different geological conditions, providing strong support for related CCS projects.
The starting point of this study is to break through the current computational bottleneck faced by CCS, find a more efficient and practical path, and help the engineering application of CCS technology.By exploring the novel applications of computer vision in the field of Carbon Capture and Storage (CCS), researchers hope to accelerate the promotion and popularization of CCS, contributing to the global effort to combat climate change.
In the coming years, the results are expected to be applied in the following areas:
Advertisement
Firstly, it can be directly used to guide the site selection and planning of CCS projects.
By inputting the images of the geological structure of candidate sites, it is possible to quickly predict the surface displacement under different schemes, allowing decision-makers of CCS projects to more efficiently screen the optimal injection point location and injection parameters.
While ensuring safety, it maximizes the amount of carbon sequestration, thereby greatly saving time and cost in site assessment.Secondly, the deep learning model developed in this study can be integrated into the CCS risk management system to achieve real-time monitoring and early warning for the injection process.
Once the monitoring data shows abnormal changes in the strata state, the deep learning model can quickly predict the response of surface displacement, providing a basis for timely countermeasures, thereby minimizing the environmental risks of CCS.
Thirdly, it can be used for public communication of CCS projects.
By utilizing intuitive visualization effects, the potential impact of CCS on the surface under different scenarios can be demonstrated, which helps to improve the public's understanding and cognition of CCS, enhance people's confidence in the safety of CCS, and promote the smooth implementation of CCS projects.
Fourthly, the methods used in this study can also be applied to other coupled fluid-solid mechanics problems, such as shale gas extraction, geothermal development, etc.The end-to-end learning paradigm driven directly by images is expected to open up new avenues for efficient numerical simulation in these fields.
Fifthly, it provides new ideas for interdisciplinary applications.
This achievement demonstrates how to combine computer vision, a cutting-edge IT technology, with traditional geological engineering problems, fully tapping into the strengths of different disciplines.
This paradigm has significant implications for solving other major challenges in the field of Earth science.
In summary, this achievement takes surface displacement prediction as a breakthrough point, providing a new breakthrough for the engineering application of CCS technology.
Please note that "CCS" is not defined in the original text. If it refers to a specific technology or concept, you may need to specify it for clarity in the translation.As research continues to delve deeper and improve, it is expected to generate tangible application value in various aspects, helping Carbon Capture and Storage (CCS) to play a greater role in mitigating global climate change.
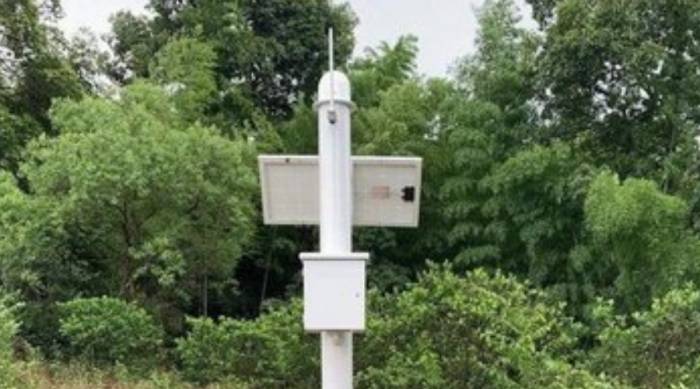
At the same time, this work also sets an example for interdisciplinary integration and collaborative innovation.
Recently, the relevant paper was published on arXiv[1] with the title "CarbonNet: How Computer Vision Plays a Role in Climate Change? Application: Learning Geomechanics from Subsurface Geometry of CCS to Mitigate Global Warming."
Stanford University Ph.D. student Wei Chen is the first author and co-corresponding author, and Stanford University Ph.D. students Yunan Li and Tianyuan are co-authors.
It is reported that as the issue of global warming becomes increasingly severe, reducing emissions of greenhouse gases such as carbon dioxide and achieving carbon neutrality has become a common mission for the whole world.CCS is widely recognized as one of the key pathways to achieve this goal. CCS effectively reduces the amount of carbon dioxide in the atmosphere by capturing carbon dioxide produced during the industrial production process and then transporting it to underground saline aquifers or depleted oil and gas fields for permanent storage.
However, the large-scale implementation of CCS still faces many challenges. One of the main risks is that the high-pressure carbon dioxide injected into the strata may cause surface uplift, which can damage ground buildings and affect people's lives.
Accurately assessing and predicting the spatiotemporal distribution characteristics of surface deformation during the CCS process is crucial for the safe and effective implementation of CCS projects.
Traditional methods mainly rely on direct numerical simulation, but due to the large spatial and temporal scales involved in CCS, detailed simulation often requires extremely high computational resources, which is costly and time-consuming, and not conducive to the planning and decision-making of actual CCS projects.
Chen Wei, Li Yunan, and Tian Yuan, three doctoral students, although belonging to different research fields, are good friends with each other.In daily communication, they realized that the CCS project is facing challenges in actual implementation, especially in the site selection evaluation phase, which requires a large amount of computing resources for numerical simulation.
The three quickly captured this pain point and decided to carry out interdisciplinary collaborative research by combining their respective strengths. Subsequently, they began to conceive the framework and steps of the entire research.
Firstly, relying on Li Yunan's research accumulation in the field of CCS, the key issues to be solved were clarified: that is, how to use deep learning technology to accelerate the site selection analysis of CCS.
At the same time, they combined this topic with the course project of Stanford University's CS231N course, making the research process complement the daily learning.
After establishing the research direction, Chen Wei and Tian Yuan were responsible for the basic theoretical issues of computational mechanics and strata displacement, laying a solid foundation for the subsequent machine learning modeling.At the same time, Li Yunan began the data collection and organization work, providing data support for training high-quality deep learning models.
Once the data was ready, the three of them started to devote themselves to the stage of model construction and training. During this period, they repeatedly conducted experiments, comparing and testing different network structures and hyperparameters, striving to find the optimal model configuration. Ultimately, they found an innovative deep learning network architecture suitable for the problem.
In the research, the problem of data acquisition became a difficult problem in front of them. In order to obtain sufficiently realistic geological data, they first went out separately, trying to seek data sharing from major energy companies and academic institutions.
However, after several twists and turns, the gains were very limited. Many data holders were reluctant to fully open the information in their hands due to privacy and confidentiality considerations.
Just when everyone was at a loss, they thought, isn't this geological data essentially the result of computational mechanics simulation?Rather than obtaining data from others, it is better to leverage one's strengths in the field of computational mechanics to generate a diverse range of simulated data.
Thinking of this, the three of them immediately took action. They collaborated to write a program to simulate various geological conditions, thereby generating a vast amount of surface displacement data.
At the same time, they also realized that although this research has already shown initial results, there is still a lot of room for further exploration and expansion. Therefore, after discussion, the three of them formulated a series of follow-up research plans.
The primary task ahead is to further improve the existing deep learning model. Although the current model can already predict surface displacement relatively accurately, they believe there is potential for optimization.
They plan to build on the existing framework and attempt to introduce more advanced network structures, such as attention mechanisms, in order to further enhance the model's performance and generalization capabilities.At the same time, they also plan to expand the input dimensions of the model, incorporating more geological and engineering parameters, so as to be applicable to more complex and variable actual scenarios.
Secondly, they also plan to expand the geographical scope of the research. The current research is mainly based on the geological conditions of a specific area, and next they hope to promote the application of the model to other typical geological structural areas, in order to test the applicability and robustness of the model.
This requires the collection and processing of more diverse data, and the fine-tuning and optimization of the model according to the characteristics of different regions.
Furthermore, they will also expand the depth and breadth of the research. In terms of depth, they plan to explore the application of deep learning technology in other complex coupled fluid-solid problems, such as shale gas extraction, geothermal development, etc.
In terms of breadth, they hope to expand the current two-dimensional model to three-dimensional space to obtain more comprehensive and refined predictive results.In addition to deepening on the technical level, they also hope to strengthen cooperation and exchange with the industry and academia. For example, by establishing a closer cooperative relationship with energy companies, applying research findings to actual CCS projects, and using practice to provide feedback and improve models.
POST A COMMENT